At the University of Vilnius in Lithuania the scientific focus of Dr. Valius Mindaugas and Nadežda Dreižė is on protein biomarkers and their characterization. Apart from biochemical methods the visualization of proteins and evaluation of microscopic images is an approach to understand the functions of the Biomarkers of interest. The topic of protein localization and chemical modification of amino acids is tackled using confocal laser scanning microscopy. (The epitopes of the respective proteins are marked with fluorescent antibodies).
Two different Research Approaches
The first approach involves working closely with physicians. Postoperative tissue material is analyzed for markers that indicate resistance. In particular, researchers screen for specific proteins which are only found in the event of resistance against certain cancer drugs. The results provide an indication whether or not a certain type of therapy will benefit a patient.
The second approach is more closely linked to live cell microscopy. The matter of investigation is the impact of selected chemical compounds on the growth and cell signaling behavior. Cell lines screened for resistances against the respective compounds are compared versus wild type cell lines as part of a before/after analysis. Different biochemical and imaging techniques are employed to explore the drugs' effect on the cancer cells.
When analyzing the cells, researchers aim to identify certain mechanisms that can be treated with anti-cancer drugs. The goal is to ascertain the exact location of the proteins in the cells and identify their functions. For the visualization of these proteins researchers use antibodies. Then, a wide range of different algorithmic approaches are used to analyze the texture of microscope images.
The researchers analyze their images with a ZEISS microscope.
The Research Process with arivis Cloud
Image processing and evaluation in general takes a lot of time because often a bundle of different software packages has to be applied. In addition, modifications in the workflows according to the respective task have to be made which require highly skilled imaging specialists. One can easily spend several days of work on these topics.
Before using APEER the identification of cells was done manually and only an unprecise estimation of the changes could be made. By using APEER the detection and quantification of phosphorylation events per single nucleus was possible.
Therefore, the outcome of an experiment is much more significant and also more precise when it comes to making a statement on the respective mechanisms and events. In APEER both control groups can be assessed in a batch mode which means that workflows are running in parallel in the cloud on a huge number of images. This makes the whole approach more time efficient and solves the problem to get a significant number of values to cover the required statistics.
Due to new features, APEER can also be easily used as a tool to share, discuss and visualize results (images, graphs and values) with collaborators or colleagues.
With arivis Cloud the image processing became more time efficient.
Technical Background
The arivis Cloud team created a special workflow for the research tasks of Prof. Mindaugas and his team from the University of Vilnius. In the first step, the images are split into their color channels using the ChannelSplitter module. Blue shows the nuclei stained with DAPI, green shows the TP53 proteins (antibodies), and red shows the Phosphorylated Ser15 of TP53 proteins. This means we have a separate picture for each color.
Detailed Description of the Modules
In the next step, we perform a segmentation of the blue channel by applying an Otsu Auto-Thresholding algorithm, the Auto Thresholding module. With the use of OpenCV, we determine the counters for each segmented object, creating a mask for the cell nuclei (Fig.1).
Fig.1: Mask for the cell nuclei
At this point, the result is not perfect. Due to overlapping cells, some of the segmented objects are clusters of cell nuclei. These clusters are removed by filtering on the object area. The number of remaining single nuclei is still enough to obtain reliable statistics. This mask is then applied to the green and the red channels to extract the mean intensities per cell nucleus. We also need to know the number of stained S15 grains per nucleus (red color). To count the S15 grains automatically, we apply a Laplacianof Gaussian Algorithm, see Fig.2. The corresponding APEER module is called the Laplacian of Gaussian Blob Detector.
Fig.2: Outcome of detecting S15 grains
Using the nuclei mask from the blue channel, we extract the number of S15 grains per nucleus. Based on these results, we compute the following measurements:
1. Ratio of red over green intensity per nucleus
2. Number of S15 grains over green intensity per nucleus
For both measurements, the histogram is plotted over all cell nuclei.
This process is done for two types of cell populations:
· Cells that are not treated with oxaliplatin
· Cells that are treated with oxaliplatin for 24h,
Analyzing the Results
The comparison of the histograms demonstrates the effect of oxaliplatin on the HCT116 cancer cells:
1. The first results show that most cells without treatment have a ratio of red over green intensity between 0.5 and 1.1, but there are also a few cells with a higher intensity level of almost 3.0.
After treating the cells with oxaliplatin, we see significant changes in the statistics. A higher number of cells have a ratio between 0.5 and 1.0, and only a few cells have a large ratio intensity. However, none is higher than 2.4.(Fig.3)
Fig.3: Results without and with treatment: red over green channel intensity
2. The second results show the number of S15 grains over green intensity per nucleus. Most of the cells without treatment have a relatively low intensity. After the treatment, the number of cells with almost no intensity is clearly lower. Also, the variety of different intensities is higher and goes up to 0.0030. (Fig.4)
Fig.4: Results without and with treatment: number of red grains in relation to green intensity
Summary of the Workflow
The whole workflow is built on APEER. All steps of the workflow are automated.
1. Input: Microscopy picture
2. Separation of the image into different color channels
3. Nuclei segmentation: cell nuclei are lifted off the background
4. Gaussian Blob Detector: counts the grains per cell nucleus
5. Result: statistics
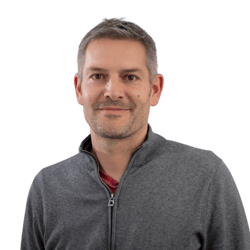